In the fast-paced world of healthcare, the accuracy and reliability of data play a crucial role in providing effective patient care, making informed decisions, and achieving better outcomes. However, data quality issues in healthcare are common and can have severe consequences.
In this blog post, we will explore in-depth what data quality in healthcare means, its requirements, the significance of maintaining high data quality, the impact of poor data quality on different aspects of healthcare, and effective strategies to improve data quality.
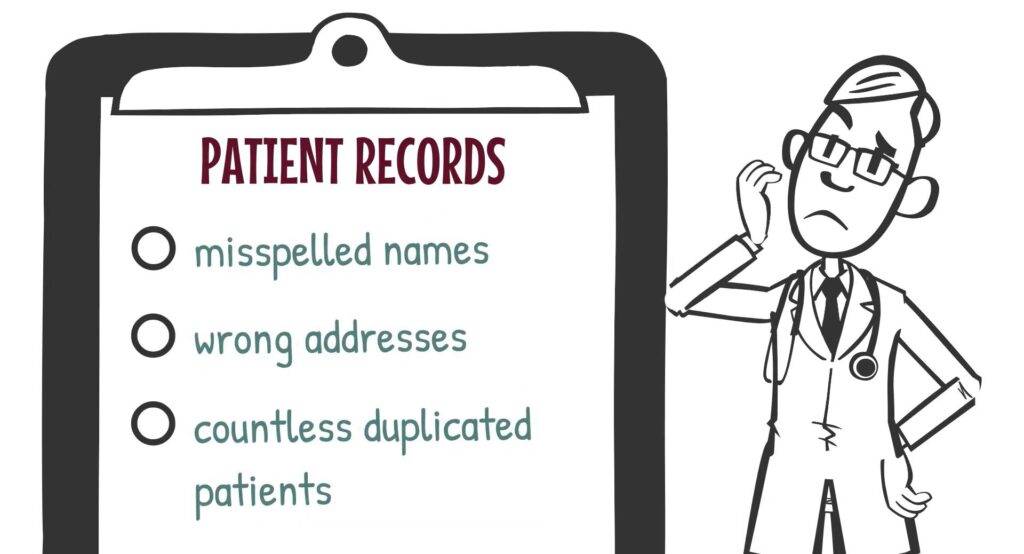
What is Data Quality in Healthcare?
In the rapidly evolving landscape of healthcare, data quality stands as a critical pillar that underpins the success and efficacy of patient care and administrative processes. Data quality in healthcare refers to the accuracy, completeness, consistency, and timeliness of the vast array of healthcare data collected from diverse sources, including electronic health records (EHRs), medical devices, insurance claims, patient surveys, and administrative databases.
This data serves as the backbone of medical decision-making, research, policy formulation, and overall healthcare management.
Accuracy
Accuracy in healthcare data is of paramount importance as it directly impacts patient safety and treatment outcomes. Inaccurate data can lead to misdiagnoses, incorrect treatment plans, and medication errors, posing severe risks to patient health. Even minor inaccuracies, such as incorrect dosage information or misspelled patient names, can result in grave consequences. To ensure data accuracy, healthcare organizations must implement robust data validation processes. Automated validation tools can detect errors and inconsistencies, flagging data for review and correction.
Completeness
Complete data refers to the availability of all relevant and essential information required for comprehensive patient care and analysis. Missing data, whether due to human error or system limitations, can hinder healthcare professionals from obtaining a holistic view of a patient’s medical history, potentially leading to incomplete diagnoses and inadequate treatment decisions. To improve data completeness, healthcare institutions should adopt standardized data entry protocols and ensure that all relevant data points are consistently captured.
Consistency
Data consistency ensures that information aligns across different sources and systems within the healthcare ecosystem. When data inconsistencies arise, they can lead to conflicting patient histories, treatment plans, and medical records, creating confusion among healthcare professionals and posing risks to patient safety. Data integration and standardization initiatives are essential to maintaining data consistency across various platforms and databases. By adopting interoperable data standards and well-defined coding systems, healthcare organizations can reduce the likelihood of discrepancies and enhance the accuracy of patient information.
Timeliness
Timely data is crucial for enabling real-time decision-making in healthcare. Rapid access to the most current patient data allows healthcare professionals to respond promptly to changing patient conditions, making well-informed decisions, and providing appropriate and timely interventions. Timeliness is particularly critical in emergency situations or when dealing with rapidly evolving health conditions. To enhance data timeliness, healthcare institutions should focus on data capture and updating processes. Implementing efficient data entry protocols and leveraging technology solutions can reduce delays in data availability.
What are Data Quality Requirements in Healthcare?
Maintaining high data quality in healthcare is a multifaceted endeavor that demands adherence to specific requirements. These essential data quality requirements form the foundation for accurate, reliable, and secure healthcare data management:
Standardization
Accuracy in healthcare data is of paramount importance as it directly impacts patient safety and treatment outcomes. Inaccurate data can lead to misdiagnoses, incorrect treatment plans, and medication errors, posing severe risks to patient health. Even minor inaccuracies, such as incorrect dosage information or misspelled patient names, can result in grave consequences. To ensure data accuracy, healthcare organizations must implement robust data validation processes. Automated validation tools can detect errors and inconsistencies, flagging data for review and correction.
Data Governance
Data governance serves as the guiding framework for establishing clear roles, responsibilities, and processes related to data management. By implementing data governance practices, healthcare institutions create a systematic approach to data quality management and ensure data is used responsibly and ethically. Assigning data stewards responsible for overseeing data quality initiatives and enforcing data governance policies is crucial. These stewards act as guardians of data integrity, advocating for data quality improvement, and driving data governance awareness throughout the organization.
Data Validation
Regular data validation is a proactive strategy that involves verifying data accuracy, completeness, and consistency through a systematic review process. Data validation can occur through various means, including manual audits, automated integrity checks, and cross-referencing data with external sources for verification. Audits and integrity checks help identify discrepancies, errors, and missing data elements, enabling timely corrections and ensuring that healthcare data is reliable and trustworthy. Implementing automated data validation tools streamlines the process, reduces human error, and enhances the efficiency of data quality assessments.
Data Security
Data security is a fundamental aspect of data quality in healthcare. Protecting patient data from breaches, unauthorized access, and cyber threats is crucial to maintaining data integrity and fostering patient trust in the healthcare system. Healthcare organizations must invest in robust cybersecurity measures, such as encryption, firewalls, and multi-factor authentication, to safeguard sensitive patient information. Additionally, staff training on data security best practices is essential to ensure that all personnel understand their roles in protecting data and are aware of potential risks and threats.
Why Data Quality is Essential for Healthcare
High-quality data serves as the lifeblood of the healthcare industry, playing a pivotal role in improving patient outcomes, enhancing decision-making processes, driving medical advancements, and ensuring compliance with stringent regulations. The significance of data quality in healthcare is evident in the following aspects:
Patient Safety
Patient safety is the foremost priority in healthcare, and accurate and reliable data forms the cornerstone of safe and effective patient care. Healthcare professionals heavily rely on precise and up-to-date patient information to make critical decisions, such as diagnosing medical conditions, devising treatment plans, and administering medications. Poor data quality can lead to medical errors, misdiagnoses, and inappropriate treatments, potentially causing harm to patients and eroding trust in the healthcare system. Ensuring data accuracy and completeness is, therefore, paramount for safeguarding patient safety.
Informed Decision-Making
Data-driven decision-making is at the heart of healthcare quality improvement initiatives. Access to timely, accurate, and comprehensive data empowers healthcare leaders, clinicians, and administrators to gain valuable insights into patient populations, clinical outcomes, and resource utilization. By analyzing trends and patterns through advanced analytics tools and data visualization dashboards, healthcare professionals can identify areas for improvement, implement evidence-based practices, and optimize healthcare delivery. Informed decision-making facilitates efficient resource allocation, reduces waste, and fosters continuous quality improvement across the healthcare ecosystem.
Research and Innovation
High-quality data serves as a catalyst for medical research and innovation. Researchers depend on accurate and comprehensive datasets to conduct clinical trials, analyze disease trends, and develop new treatments and medical technologies. Robust data sharing and collaboration among healthcare institutions, research organizations, and industry stakeholders expedite scientific discoveries and enable medical breakthroughs. By leveraging high-quality data, researchers can identify novel approaches to disease management, uncover personalized treatment options, and improve overall healthcare practices.
Regulatory Compliance
The healthcare industry is subject to a myriad of regulations and standards, with data privacy and security being of utmost importance. Compliance with stringent regulations, such as the Health Insurance Portability and Accountability Act (HIPAA), is vital for protecting patient privacy and maintaining public trust. Non-compliance with data quality and security requirements can lead to severe consequences, including legal penalties, reputational damage, and loss of patient confidence. Healthcare organizations must prioritize data privacy and compliance efforts, implement robust data security measures, and ensure data integrity to adhere to regulatory mandates.
The Impact of Poor Data Quality in Healthcare
Patient Frustration and Mistreatment
Poor data quality in healthcare can have profound implications for patient well-being and satisfaction. When data is inaccurate, incomplete, or inconsistent, it can lead to incorrect diagnoses, inappropriate treatments, and unnecessary medical tests. Such mistakes can cause frustration and confusion among patients, who rightfully expect accurate and personalized care. Moreover, mistreatment resulting from poor data quality can lead to avoidable health complications and adverse outcomes, eroding patient trust in the healthcare system.
To address patient frustration and mistreatment due to data quality issues, healthcare providers must prioritize data accuracy and completeness. Regular data quality assessments and audits can identify areas for improvement and facilitate data corrections. Involving patients in their care by encouraging them to review and verify their medical records can foster transparency and mutual trust between patients and healthcare professionals. Additionally, healthcare organizations should invest in patient engagement initiatives that empower individuals to participate actively in their care plans and contribute to data accuracy by providing relevant information.
Employee Frustration and Distrust
Data plays a pivotal role in guiding healthcare professionals’ decision-making processes. When data quality is compromised, healthcare providers may find themselves uncertain about the accuracy of the information they rely on. Inconsistent or unreliable data can create hesitancy and doubt among staff members, leading to a sense of frustration and distrust in the data-driven decision-making approach.
To address employee frustration and distrust related to data quality, healthcare organizations must emphasize the importance of data governance and quality. Providing data quality training to employees can equip them with the skills and knowledge needed to identify and address data quality issues. Involving staff in data improvement initiatives and soliciting their feedback on data usability and accuracy can foster a culture of data-driven excellence. Leadership support for data governance and continuous improvement efforts can also boost employee confidence in the data and reinforce its significance in delivering high-quality patient care.
Employee Inefficiency
Inaccurate or incomplete data can lead to significant inefficiencies in healthcare workflows. Healthcare professionals may spend valuable time cross-referencing data from various sources, attempting to correct errors, and reconciling conflicting information. This redundancy and data-related inefficiency divert attention away from patient care and impede productivity.
To enhance employee efficiency, healthcare organizations must streamline data entry processes and implement user-friendly electronic health record (EHR) systems. Automated data validation tools can perform real-time checks, flagging potential errors during data entry and reducing the likelihood of inaccuracies. By promoting interoperability among different systems, healthcare professionals can access comprehensive patient information in a cohesive manner, minimizing the need for redundant data verification. Additionally, empowering staff with time-saving tools and technologies can optimize their productivity and allow them to focus on delivering quality patient care.
Poor Policy Decisions
Data-driven policy decisions are only as reliable as the data they are based on. When data quality is compromised, policy-makers may make misinformed decisions that have far-reaching consequences on the healthcare system’s efficiency and effectiveness. Inaccurate data can lead to misinterpretations, biases, and suboptimal resource allocation, ultimately hindering progress toward better healthcare outcomes.
To prevent poor policy decisions resulting from data quality issues, healthcare leaders must establish robust data governance frameworks. These frameworks should include well-defined data quality metrics to assess and monitor the accuracy, completeness, and consistency of data. Regular data quality audits can identify areas where data quality falls short, guiding improvements and ensuring the reliability of data used for policy-making. Collaborating with researchers and experts in healthcare data analysis can also provide valuable insights, validating data interpretations and supporting evidence-based policy decisions.
How to Improve Data Quality in Healthcare
Integrated Data Analytics
Implementing integrated data analytics solutions represents a transformative approach to enhancing data quality in healthcare. By consolidating data from disparate sources, such as EHRs, laboratory systems, and administrative databases, healthcare organizations can create a unified and comprehensive view of patient information. This data integration fosters data standardization, ensuring that data elements are consistently represented across different systems, reducing redundancy, and eliminating data silos.
Furthermore, integrated data analytics enable healthcare professionals to leverage advanced analytical tools and machine learning algorithms to identify patterns, trends, and potential data quality issues. These tools can perform data profiling and anomaly detection, flagging potential errors, inconsistencies, or missing data elements. Timely interventions can be made to rectify data quality issues and prevent their escalation.
Regular data audits and quality assessments are indispensable in maintaining data reliability. By conducting routine data audits, healthcare organizations can verify data accuracy, completeness, and consistency. Audits can also reveal potential weaknesses in data capture processes, identify areas for improvement, and drive data quality initiatives.
Using the Correct Tools and Metrics
Selecting the right data management tools is essential for effective data quality improvement. Data profiling tools, data validation software, and data quality dashboards can streamline the data quality assessment process and minimize manual errors. Automated data validation tools can perform data integrity checks, cross-referencing data against predefined rules, and external data sources. These tools can significantly reduce the time and effort required for data validation, allowing healthcare professionals to focus on data-driven decision-making and patient care.
Defining appropriate data quality metrics is crucial for measuring and monitoring data quality improvements over time. Metrics such as data completeness percentages, accuracy rates, and data consistency scores provide quantifiable measures of data quality. By regularly tracking these metrics, healthcare organizations can assess the impact of data quality initiatives and identify areas that require further attention.
Using a Suitable Format
Standardizing data formats and data entry protocols is vital for ensuring data consistency and minimizing errors in data collection. By adopting standardized data entry templates and following data coding guidelines, healthcare staff can ensure that data is recorded uniformly across the organization. Regular training sessions on data entry procedures and data quality best practices can further reinforce the importance of data accuracy and completeness among healthcare professionals.
Implementing data validation rules during data entry can serve as an immediate quality control mechanism. These validation rules can prevent incomplete or inaccurate information from being recorded, reducing the likelihood of data errors and discrepancies. Real-time data validation alerts can prompt healthcare professionals to review and correct data errors at the point of data entry, improving data quality and accuracy.
Solve Data Quality Issues in Healthcare With 4medica
At 4medica, we recognize the utmost importance of data quality in healthcare services. With a steadfast commitment to accuracy, completeness, and reliability, our integrated data analytics solutions and data governance frameworks empower healthcare organizations to deliver exceptional patient care and make informed decisions.
Through streamlining workflows, fostering a data-driven culture, and ensuring precision in data, we stand dedicated to elevating the healthcare landscape through data-driven excellence. Together, we build a future where data quality remains at the core of healthcare success, advancing patient outcomes and transforming the industry for the better.
Contact us today to get started.